Artificial Intelligence in Molecular imaging
Over the last years, the quality of (endoscopic) imaging has increased. The introduction of molecular imaging adds to this increase and to further enhance this modality we are introducing computer-aided software based on artificial intelligence (AI) in endoscopic imaging in the gastrointestinal (GI) tract.
AI in endoscopy
Through the introduction of molecular imaging in gastrointestinal endoscopy, the quality of the endoscopic assessment of the GI tract is enhanced. In order to fully exploit the functionality of fluorescent molecular endoscopy (or other advanced endoscopy techniques), training and experience of the endoscopist is required to understand the fluorescent signals of the targeted biomarkers. To alleviate the burden of training and experience and to facilitate potential implementation of molecular imaging in future clinical practice, AI could be used to support endoscopists in the real-time interpretation of molecular imaging. By building mathematical models based on given data, predictions can be done about new, unseen data. The model needs dedicated data to be trained, and the more data is used for training, the better the expected performance of the algorithm is. Two forms of AI can be distinguished in GI endoscopy: computer-aided detection (CAD) and computer-aided diagnosis (CADx) algorithms. The first can aid in red-flagging a lesion during examination, the latter for further classification of the detected pathology. Both forms of AI could be applied in FME
Focus
In our first collaborative study with Fons van der Sommen, part of the center for care & cure technology Eindhoven, we investigated whether AI as a second assessor can support gastroenterologists in interpreting endocytoscopy (EC) images of the Barrett’s esophagus. EC is an ultra-magnification technique that enables visualization of the tissue histology but subsequently produces rather complex endoscopic images. Through our data, we observed that AI-assisted gastroenterologists outperformed gastroenterologists without AI support by achieving sensitivities of approximately 95%. Thus, the best sensitivities emerged through man-machine collaboration and resulted in the best dysplasia identification in complex endoscopic images. Therefore, AI as second assessor could support clinical implementation of advanced endoscopy techniques such as molecular imaging. Which is why we plan to conduct studies with AI in fluorescence molecular endoscopy.
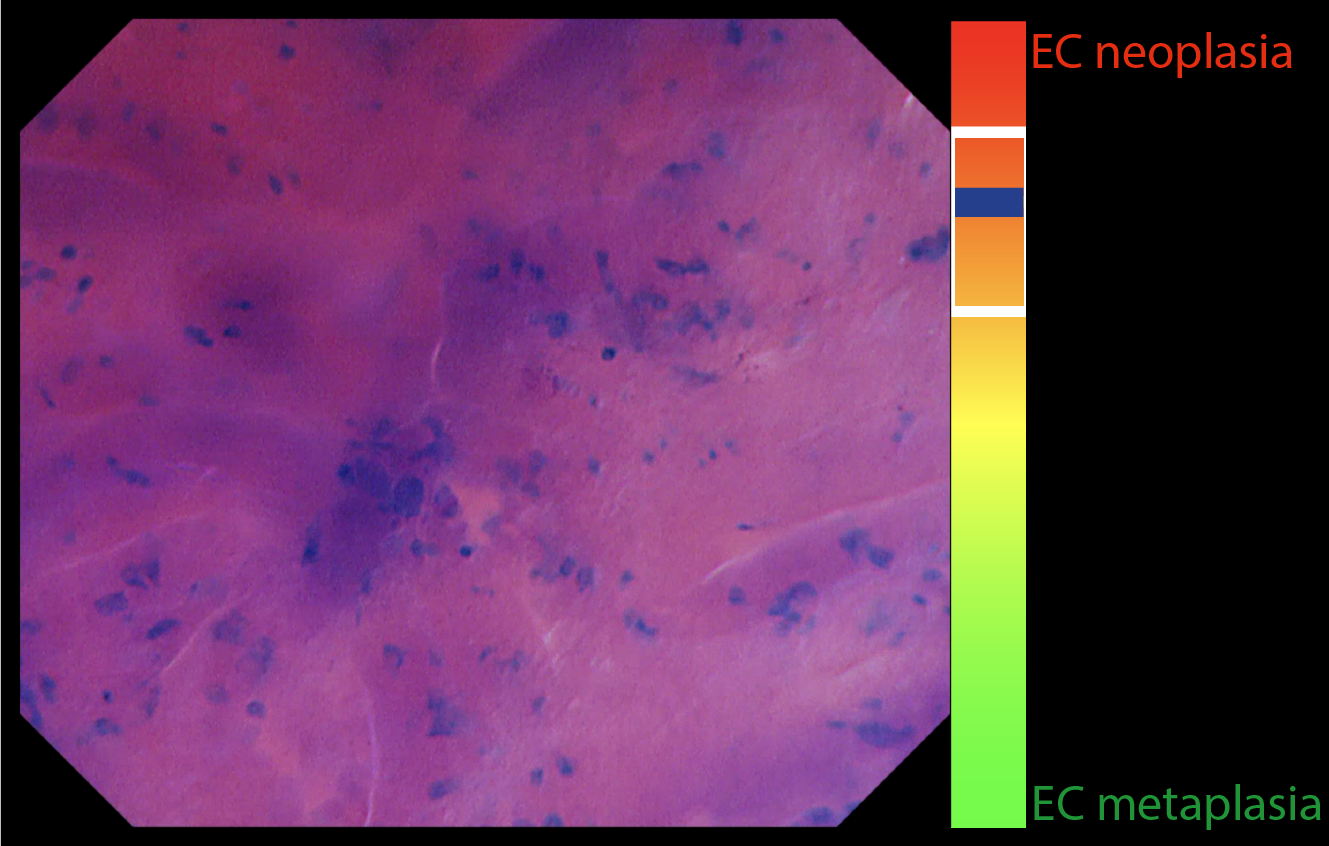